Summary Introduction: After AlphaGo beat Li Shishi, the scenery of deep learning is no longer there. The deep learning technology based on artificial neural network shows the intelligence of the machine with its black box "thinking" mode - &mdash...
Guide: After AlphaGo beat Li Shishi, the scenery of deep learning is no longer there. The deep learning technology based on artificial neural network shows the intelligence of the machine - a kind of "intuition" ability with its black box "thinking" mode. However, the "intuition" of the machine can win on Go, which means that it can also change the field to display "talent" to solve problems such as seeing a doctor? If the answer is no, does that mean that machine intelligence is just the same thing, for example, if the level of chess is higher, the machine can't do it "deliberately lose"? This article tells you an unexpected answer. Go to AlphaGo four to one victory over Li Shishi, amazing degree of technological progress. On the other hand, the disk that the machine lost caused a variety of suspicions, and even the conspiracy theories of "deliberate release of water" came out. There are also people who understand machine learning in a small voice: Isn't Li Shishi's "one hand of God" exactly the anti-sample of AlphaGo?
In the past few years, deep learning has become purple, but it is not invulnerable. "Competing with the sample" is an unclear "hidden illness." There is a picture of the truth: Let's take a look at what's in the picture below.
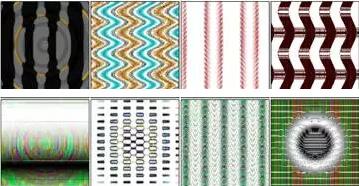
Such pictures are intentionally created to fool the learning process. They are called "combat samples."
Introduce the source before revealing the mystery. One of the most successful applications of deep learning is image recognition, which is to say what a picture depicts. After training in more than one million sample images, the deep neural network can correctly identify the things in most pictures. But the above row of pictures are identified as king penguins, starfish, baseball, electric guitars, and the next row of photos are identified as train cars, remote controls, peacocks, African grey parrots. Details of this study can be found in reference [1]. Because such pictures are intentionally created to fool the learning process, they are called "combat samples." The large number of anti-samples has been confirmed by many research institutes, but the reasons for the traps in the learning process are still different. Below is my personal analysis of this issue. Computer "intuition"
Is there a superhuman intelligence in the computer? In the article, I said that machine learning usually regards "learning" as "calculation" as a way and goal. From a computational point of view, solving a problem with a computer is to implement a function from input to output. Taking a numerical function as an example, if it is known that the relationship from input X, Y to output Z is Z = X2 - Y, then when the input is (2, 3), the output is 1; when the input is (4, 7), The output is 9; when the input is (3, 5), the output is 4. As long as the formula or algorithm that converts the input into an output is known, a program can always be programmed to complete the calculation. This is why "as long as people can explain the solution clearly, the computer can solve the problem." Artificial intelligence can be said to be a study of how to solve problems with computers when it is impossible to explain the solution. Machine learning will regard the above problem as "function fitting", that is, the general formula is unknown, only know the specific "sample" f(2,3) = 1, f(4,7) = 9 and so on, It is necessary to estimate what f(3,5) is equal to.
In the history of artificial intelligence, there have been attempts to solve such problems with the "guess function" approach, and claimed to rediscover Ohm's law and Kepler's law. The problem with this path is that there are many functions that satisfy the condition, and the samples often contain errors, so the degree of fit is not necessarily good. Now the mainstream approach to machine learning is not a "guess function" but a "catch function", that is, using a general method to create an approximation function based on the sample. Since the purpose is to use this function to deal with new problems like f(3,5), is it true that f itself can be written as a simple formula? The function is built by generalizing the sample to a similar input. Since input (3,5) as a point is just between (2,3) and (4,7), it may be considered that f(3,5) is the average of f(2,3) and f(4,7)5 . Since the "correct formula" does not exist, this prediction is completely reasonable. As to whether it is in line with the facts, it will be known after the actual inspection.
Artificial neural networks are a common "function fitter." Many people, as the name implies, believe that artificial neural networks are “working like human brainsâ€. In fact, its design is only inspired by the human brain, and its real attraction comes from its plasticity, that is, it can be changed by adjusting internal parameters. Kind of function. The initial parameters are arbitrary, so it is possible to get f(2,3) = 0. The correct answer 1 provided by the sample at this time will be used by the learning algorithm to adjust the network parameters to achieve the desired output (not necessarily exactly 1, but close enough). Next, train f(4,7) = 9 in the same way. The trouble here is that the parameter adjustment caused by the subsequent samples will destroy the processing result of the previous sample, so it must be returned after all the samples are processed. This "training" process is usually repeated many times to allow the same set of network parameters to meet all sample requirements simultaneously (as far as possible).
Understand this training process, you can see that AlphaGo can't actually sum up the lessons of the previous game through the rewinding of people, and immediately use it in the latter game, but need to put a lot of (or even all) previous samples. Go through it all at once (or even more times) to ensure that the knowledge learned from this game will not ruin the previous results. If human learning is generally "incremental local modification" (what went wrong), then deep learning is "global modification of the total amount" (all parameters are determined by the sample population).
Can deep learning promise DeepMind to enter the future of healthcare?
The knowledge gained by an artificial neural network through training is distributed among all its network parameters, which leads to the incomprehensibility of its results. AlphaGo takes a certain step at a certain moment, which is determined by thousands of parameters in the system, and the values ​​of these parameters are the product of the entire training history of the system. Even if we can really reproduce its complete history, it is often impossible to describe the "cause" of one of its decisions in a concept we can understand. There is a view that this means that the machine has a "intuition" successfully, but this needs to be viewed in two ways. Compared with the traditional computing system, it is natural to summarize a certain law from a large number of samples, but it is not an advantage to say that this law cannot be said. Many of the beliefs of mankind are so complicated that they cannot be clarified by themselves, so they are called “intuitionâ€, but not all beliefs are unclear.
Deep learning, so that the entire mainstream machine learning, to a large extent accept this kind of learning system as a "black box", the name of the "end-to-end" learning, meaning "I just want to achieve you The function is fine, you control how I do it." This method naturally has its advantages (more peace of mind), but once something goes wrong, it is difficult to tell what is going on, and it is even harder to make corresponding improvements. AlphaGo's fainting and the above confrontational samples are examples of this.
So what is the problem? It depends on the application area. For Go, I think that the program is more than a human being, and the outcome of individual matches has nothing to do with the general trend. Even if the program does have a dead hole similar to the anti-sample, it is not easy for the player to use it in the game, but it may be due to luck. The confrontational samples now found, as shown in reference [1], were created with a large amount of computation using another learning technique, the "genetic algorithm." Whether this method can be used for Go is still a problem. On the other hand, the chess path of the program is difficult to understand or imitate, which is naturally a pity for the chess world, but does not affect its winning percentage. So these questions are not fatal to the Go program.
Now that AlphaGo developer DeepMind claims to enter the medical field, that's another matter. If a machine diagnostic system collects the information directly after you have collected information about you, the whole reason is "this is my instinct", can you accept it? Of course, the system's past success rate may be as high as 95%, although it has also given athletes a headache. But the question is how do you know that you are not that 5%? Moreover, the statistical diagnosis based on big data is very effective for common diseases and frequently-occurring diseases, but the processing ability for special cases is not guaranteed, so the success rate is probably not 95%. Because of this problem, the basic assumptions and frameworks for learning the shutdown device are not solved by modifying the details of the algorithm. DeepMind may have a different path to hope.
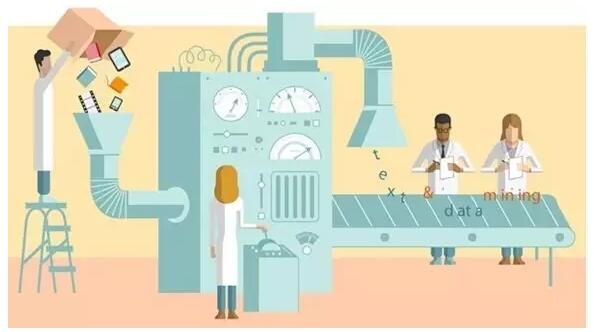
Heaven and earth outside the "black box" technology
At present, the industry's understanding of the limitations of deep learning is seriously inadequate, and the victory of AlphaGo has caused the illusion that deep learning can solve various learning problems. In fact, the academic attitude of deep learning in the past two years has changed from excited pursuit to calm examination, and the leaders of deep learning have also come forward to deny that "this technology has solved the core problem of artificial intelligence."
Seeing this, some readers may be prepared to write the following comment: "I have said it long ago, artificial intelligence is just some programs, how can you really learn like people!" - Sorry, you really said it was early. The problems mentioned above exist in deep learning, artificial neural networks, and machine learning, but the conclusions cannot be extended to the entire artificial intelligence field, because not all learning techniques are function fitting.
The solution to a problem is always made up of a series of steps. The traditional computing system allows the designer to specify both the individual steps and the entire process, thus ensuring the reliability and comprehensibility of the solution, but the system is not flexible. To a large extent, the basic goal of artificial intelligence is to give the system flexibility and adaptability. Of course, the designer is not likely to be limited. The method of function fitting can be said to "limit both ends and release the middle", that is, to constrain the input-output relationship of the system in the form of training samples, but allow the learning algorithm to relatively freely choose the intermediate steps and non-samples to realize this relationship. Processing results. Another possibility, on the contrary, can be said to be "restricting steps, letting go of the process", and my own work falls into this category.
Is there a superhuman intelligence in the computer? In the article, I mentioned the “Nas†system I designed (see Reference [2] for details). Nath is an inference system in which each basic step follows a generalized inference rule, including deduction, induction, attribution, instantiation, correction, selection, comparison, analogy, merging, decomposition, transformation, derivation, decision making, and so on. These inference rules also implement the learning function, and can flexibly connect to each other to complete complex tasks. The basic assumption here is that any thought process can be broken down into these basic steps, and “learning†is the “self-organizing†process in which the system uses these rules to build and adjust beliefs and conceptual systems with experience as raw materials.
Understanding "learning" as "self-organization of beliefs and concepts" is closer to the human learning process than understanding "function fitting", and avoids many problems in current machine learning. For example, because each step of the system follows a certain rule, the result is better interpretable. The results of artificial neural networks are often only explained in the mathematical sense, that is, "this conclusion is determined by all existing network parameters", and the conclusion of Nath generally has a logical interpretation at the conceptual level, that is, "this conclusion is from certain "The premise was introduced by a certain rule." Although the source of a belief is extremely complex, it is said to be "intuition."
The difficulty in designing a learning system such as Nash is to determine the rationality and completeness of the rules, and to balance flexibility and certainty in applying these rules. I will introduce these issues in the future. What I want to say now is that since the system is to learn by itself, it is inevitable to make mistakes, but this cannot be used as a shield against criticism. The key question here is whether these errors are understandable and whether the system can learn from mistakes to avoid repeating the same mistakes.
The anti-sample is a big problem, not only because these samples lead to misjudgment of the system (people also misjudge when recognizing pictures), but because they reveal that machine learning is in the process of "catch up functions". The intermediate results generated are fundamentally different from the layer-by-layer abstract results in the human perception process. The intermediate results of people in identifying "penguins", "peacocks" and "parrots" generally include the recognition of the general characteristics of birds such as the head, body and wings, while the features extracted by machine learning algorithms generally have no independent meaning. It only contributes to the entire "end-to-end" fitting process (increasing the correct rate, convergence rate, etc.). Therefore, if a sample is very different from the training sample, these intermediate results may lead to inexplicable output. It is difficult for the system to effectively learn from this mistake, because no one can say which step it is wrong. To avoid this problem, it is not enough to limit the input-output relationship. The intermediate result must also have the meaning of not relying entirely on the network parameters. Therefore, it is not only the mathematical characteristics of the learning algorithm, but the cognitive function. The framework is better than the black box model.
Finally, talk about the problems exposed in the comments of the Go war. As mentioned earlier, “deep learning†is overestimated as a whole, but “artificial intelligence†is underestimated. For example, some comments are half-jokingly said: "When you can win the game, you will lose intelligence, and this is something that computers can never do." As everyone knows, although AlphaGo does not do this, this feature is not technically a problem at all. There are multiple targets in a common intelligent system, and the system must weigh the impact of the action on each of the relevant targets before taking an action. In this case, the best overall action may sacrifice some secondary goals. If such a system feels that losing a game of winning can achieve a more important goal (such as increasing the ratings of subsequent games), then it is entirely possible to do so. This kind of function has already been realized in the general intelligent system including Nath, but it has not appeared in the application technology (the related issues such as "the computer should not lie" are not discussed here). Since the results of this Go game are beyond the expectations of the overwhelming majority of people, I hope that those who still have the ability to learn can use this as a guide. In the future, don’t just say that because you don’t know how to let the computer do something, then assert that it is It is impossible to do.
references
[1]Anh Nguyen, Jason Yosinski, Jeff Clune, Deep neural networks are easily fooled: High confidence predictions for unrecognizable images, IEEE Conference on Computer Vision and Pattern Recognition, 2015
[2] Pei Wang, Rigid Flexibility: The Logic of Intelligence, Springer, 2006
Our Natural Round Placemats will add a gorgeous eco - chic backdrop to your table setting . Our skilled artisans transformed naturally harvested corn husk into the intricate woven designs that makes these placemats absolute treasures for your table . They are perfect for indoor and outdoor use , and they are versatile and easy to clean .
Crochet Placemats,Crochet Table Mat,Natural Woven Placemats,Crochet Placemat Patterns
Shandong Guyi Crafts Co.,Ltd , https://www.guyicrafts.com